Bitterlich sampling
Contents |
Introduction to angle count sampling
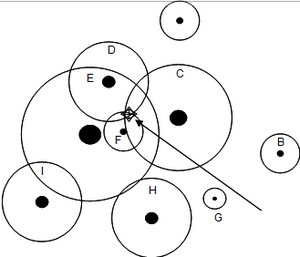
Angle count sampling was developed by Walter Bitterlich (1948), an Austrian forester. It is some times also referred to point sampling, horizontal point sampling, variable plot sampling, angle count technique, prism cruising, angle gauge sampling, and simply Bitterlich sampling.
The idea to employ nested sub-plots was introduced because we wished to have a balanced number of trees in all dimension classes; that is, we wanted to assign a higher probability of selection to the larger trees of which there are usually less in a stand (see also sampling with unequal selection probabilities).
For nested sub-plots, we defined a number of fixed plot areas in which the different dimension classes are observed. One may now further pursue that idea and develop a plot design in which the inclusion probability is strictly proportional to dimension. That is each tree has its particular and own plot size (and therefore also its particular inclusion zone). This is exactly what Bitterlich sampling does: from a selected sample point, the neighboring trees are selected strictly proportional to their basal area.
While this sounds complicated, the technique itself is very simple; the only device one needs is one that produced a defined opening angle. That can be a dendrometer, a relascope or simply your own thumb. While standing on the sample point and aiming over e.g. the thumb at the stretched out arm to the dbhs of the surrounding trees; you sweep around 360° and count all trees that appear larger than your thumb. It is obvious then, that larger trees have a larger probability of being taken as sample tree. From this counting alone you can produce then an estimate of basal area per hectare.
The only additional information that you need is the “calibration factor” of your measurement device, as, obviously, the number of trees counted depends on the opening angle which is produced by the instrument (in this case by your arm length and thumb width).
This calibration factor is also called basal area factor. Figure 1 illustrates that approach. Around each tree the individual inclusion zone is drawn. Sample points that fall into this inclusion zone will lead to an inclusion of this particular tree as sample tree. We may also imagine that these specific circular inclusion zones are concentrically placed around the sample point; then we may view them as a set of nested sample sub-plots, one for each sample tree.
The principle of Bitterlich sampling
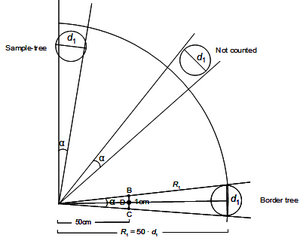
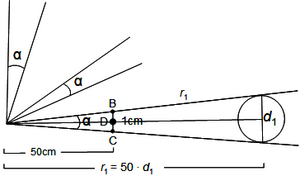
In a first step of developing this technique we need to calculate the size of these tree‑specific virtual sample plots. For each tree it holds the statement that it is only counted if it appears at 1.3 m larger than the opening angle of the measurement device. For each tree there are exactly three different situations as depicted in Figure 2: (1) either the tree is larger than the opening angle; then the tree is inside the virtual nested sub-plot and is counted; or (2) the tree is smaller than the opening angle (that is: completely covered by the thumb); that is a non-tally tree which is outside the virtual plot; or (3) the tree is exactly covered by the opening angle; then, the tree is exactly on the perimeter line of the virtual circular plot and the distance from the sample point to the center of that tree is (for practical purposes sufficiently good approximation to) the radius of the virtual circular plot. We need to find this radius, because the virtual plot will allow us to calculate the tree-specific expansion factor that we need to expand the per-plot observation to the per-hectare observation.
The opening angle can be produced by means of a stick of length c and a horizontal panel of width 1 cm at the end (analogous to arm and thumb, for example). For the virtual circle plot, for which this angle covers exactly a tree of dbh \(d_i\) (Figure 2) as a borderline tree, we obtain (approximately) plot radius \(r\) and plot area \(F_i\) along:
\[\frac{d_i}{r_i}=\frac{1}{c}\]
and then
\[r=cd_i\,\];
\[F_i={\pi}r^2={\pi}c^2{d_i}^2.\,\]
This is then the virtual plot in which all trees with exactly the dbh \(d_i\) are tallied.
This corresponds exactly to the procedure with nested circular plots where also only a defined size class was observed in each nested sub-plot. If we count \(n_i\) trees with exactly the diameter \(d_i\) (or in diameter class \(d_i\) in case that we work with diameter classes) at a sample point, then these trees have the basal area
\[{n_i}{g_i}=n_i\frac{\pi}{4}{d_i}^2.\,\]
Now, let’s expand this per-plot observation to a per-hectare figure (referring to trees with dbh \(d_i\) only!): the expansion factor is \(10000/F_i\), as usual, where \(F_i\) is the area of the virtual plot. The basal area per hectare for the trees of diameter \(d_i\) is then
\[G_{iHa}=\frac{10000}{F_i}n_i\frac{\pi}{4}{d_i}^2=\frac{10000}{\pi^2{d_i}^2}n_i\frac{\pi}{4}{d_i}^2=\frac{2500}{c^2}n_i.\,\]
Subsequently, this is done for all dbh-”classes” \(d_i\) and summed up to produce the estimate for the total basal area per hectare comprising all \(d\) classes:
\[G_{iHa}=\sum_{i}G_i=\frac{2500}{c^2}\sum_{i}n_i=\frac{2500}{c^2}N;\,\]
where \(G_{Ha}\) = basal area per hectare of all trees. The only variable in that equation is
\[N=\sum_{}n_i,\,\]
the number of observed/counted trees on the plot (over all diameter classes). This number of counted trees is multiplied with the factor
\[\frac{2500}{c^2}\,\]
which converts eventually the mere count into a basal-area-per-hectare value. This factor is called basal area factor and commonly denoted with \(k\). It is a factor that depends exclusively on the opening angle which is used.
By simply counting the \(N\) trees that are wider than our opening angle we can therefore produce an observation of basal area per hectare from a sample point:
\[G_{Ha}=k*N.\,\]
It must be observed, however, that only basal area per hectare can be observed as simple as that. For all other variable that may be of interest (such as mean diameter or number of stems), in addition the dbhs of all trees need to be measured. That is explained further below.
Basal area factors can be different. Table 1 gives the lengths \(c\) of this stick for basal area factors of 1,2,3 and 4 when there is a panel of 1 cm at the end of a that stick. Following the above formula, the length of that stick is calculated by
\[c=\frac{50}{\sqrt{k}}.\,\]
Table 1 Length \(c\) of the stick with 1cm for different
values of the basal area factor \(k\)Basal area factor (\(m^2/ha\)) c-value \(k=1m^2\,\) \(50\,\) \(k=2m^2\,\) \(35.4\,\) \(k=3m^2\,\) \(28.9\,\) \(k=4m^2\,\) \(25.0\,\)
Exact solution
Inclusion probabilities and estimators for Bitterlich sampling
For the inclusion zone approach where for each tree an inclusion zone is defined, see the corresponding article Infinite population approach. If used,the inclusion probability is then proportional to the size of this inclusion zone – which actually defines the probability that the correspondent tree is included in a sample.
We saw, for example, that angle count sampling (Bitterlich sampling) selects the trees with a probability proportional to their basal area and we emphasized that this fact makes Bitterlich sampling so efficient for basal area estimation. In contrast, point to tree distance sampling, or k-tree sampling, has inclusion zones that do not depend on any individual tree characteristic but only on the spatial arrangement of the neighboring trees; therefore, point-to tree distance sampling is not particularly precise for any tree characteristic.
In Bitterlich sampling, the selection probability of a particular tree i results from the inclusion zone Fi and the size of the reference area, for example the hectare
\[\pi_i = \frac {F_i}{10000}\]
with the Horvitz-Thompson estimator, we have the total
\[\hat \tau = \sum_{i=1}^m \frac {y_i}{\pi_i}\]
for any tree attribute \(y_i\). Applied to estimating basal area \(y_i = g_i = \frac {\pi}{4} d_i^2\) and its per hectare estimation, we have
\[\hat \tau = \sum_{i=1}^m \frac {y_i}{\pi_i} = \sum_{i=1}^m \cfrac {\cfrac {\pi}{4} d_i^2}{\cfrac {F_i}{10000}}\]
and with \( F_i = \pi r_i^2 = \pi c^2 \, d_i^2\), we have the same as Bitterlich sampling
\[\hat \tau = \sum_{i=1}^m \frac {y_i}{\pi_i} = \sum_{i=1}^m \cfrac {\cfrac {\pi}{4} d_i^2}{\cfrac {\pi c^2 \, d_i^2}{10000}} = \frac {2500 \pi}{\pi c^2} \sum_{i=1}^m \frac {d_i^2}{d_i^2} = \frac {2500}{c^2} m\]
which is the estimated basal area per hectare from one sample point where m trees were tallied. The factor 2500/c² is the basal area factor, for details see Bitterlich sampling.
References
- ↑ 1.0 1.1 Kleinn, C. 2007. Lecture Notes for the Teaching Module Forest Inventory. Department of Forest Inventory and Remote Sensing. Faculty of Forest Science and Forest Ecology, Georg-August-Universität Göttingen. 164 S.
- ↑ Kramer H. and A. Akca. 1995. Leitfaden zur Waldmesslehre. 3rd edition. J.D. Sauerländers Verlag, Frankfurt. 266p.