Cluster sampling examples
![]() |
Languages: |
English |
Example 1:
Assume that a study with relatively large square sample plots of 50 m x 50 m had been carried out, on which all tree positions were mapped. Because the individual plots were relatively large, there were only resources available to measure \(n=10\) sample plots. The small sample size led to a fairly high value of the estimated error variance.
A colleague of yours suggests: As you have mapped all trees on your 50 m x 50 m plot, you can easily make four plots of 25 m x 25 m out of each original plot. By that, you increase the sample size to the fourfold and, thus, reduce the error variance.
Does this suggestion seem reasonable?
Of course not!! The sampling design and the randomization scheme applied define what the independent observation unit is. In this case, each one of the 50 m x 50 m plots had been selected randomly and constitutes therefore one independent observation. This one single independent observation cannot be subdivided into more independent observations; it is just one. The sub-division may help learning about the spatial distribution of the variable of interest within the clusters and may, therefore, be very instructive for the optimization of the cluster plot design – but it does not help reducing the error variance!
Example 2:
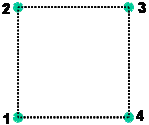
In a regional forest inventory in Northern Costa Rica, cluster plots of four sub-plots were used (as showed in figure 1), arranged at the corners of a square of 150 m side length. A sample of around 900 cluster plots was systematically laid out over the region of interest. At the center point of each sub-plot one may observe the dichotomous variable “forest-or-not” in order to produce an estimation of forest area.
At each one of the four sub-plots (points) the observation “1” (forest) or “0” (non-forest) is made so that for an entire cluster plot there are the following five possible values, depending how many sub-plots come to lie in forest: 0.0, 0.25, 0.5, 0.75 and 1.0. Table 1 gives summary statistics that are required for different approaches to area estimation and Table 17 gives the resukts for three approaches.
Table 1 Summary datas required for area estimation from cluster plots as of figure 1 (Kleinn 1993).
Data (inventory Zona Norte of Costa Rica)
(Kleinn 1993)Sample size of clusters \(n\) 899 Clusters with subplot no. 1 in forest 203 Clusters with forest subplots 282 Clusters with 1 forest subplot 52 Clusters with 2 forest subplot 60 Clusters with 3 forest subplot 58 Clusters with 4 forest subplot 114 Clusters without forest plots 617
References
- ↑ Kleinn C. 1993: Single tree volume estimation with multiple measurements using importance sampling and control variate sampling - an empirical study. IUFRO Conference on Modern Methods of Estimating Tree and Log Volume and Increment, June 14-16, 1993, Morgantown, West Virginia, USA.
- 2. Kleinn, C. 2007. Lecture Notes for the Teaching Module Forest Inventory. Department of Forest Inventory and Remote Sensing. Faculty of Forest Science and Forest Ecology, Georg-August-Universität Göttingen. 164 S.
![]() |
sorry: |
This section is still under construction! This article was last modified on 12/16/2010. If you have comments please use the Discussion page or contribute to the article! |